个人信息
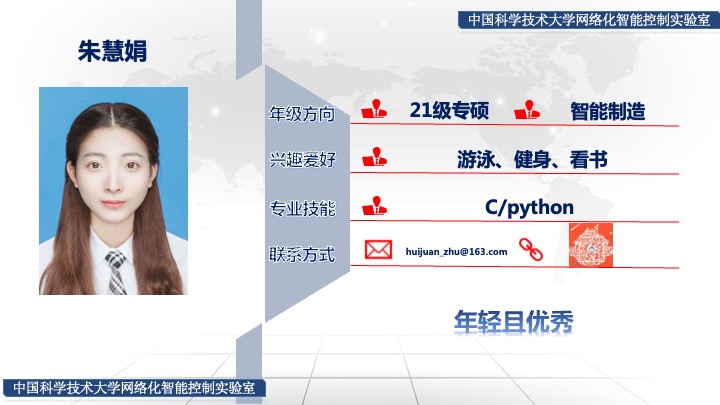
参与实验室科研项目
人机智能协同关键技术及其在智能制造中的应用
非可信智能驱动的可靠智造
学术成果
共撰写/参与撰写专利 4 项,录用/发表论文 3 篇,投出待录用论文0篇。 联培学生可能有其他不在此展示的论文/专利。
patent
-
基于跨模态生成式学习的液压减震器设备异常检测方法
赵云波,
朱慧娟,
闫晓辉,
and 康宇
2023
[pdf]
-
锡膏印刷机在线故障预测软件V1.0
赵云波,
陈龙鑫,
朱慧娟,
康宇,
and 许镇义
2022
[Abs]
[pdf]
本软件系统用于锡膏印刷机的在线故障预测,通过 url 接口实时获取锡膏印刷机 的状态数据,调用事先训练好的机器学习模型进行故障预测,并将故障预测结果 展示在前端可视化界面,以辅助工程师及时进行维护。
-
故障诊断模型的训练方法、装置、电子设备及存储介质
赵云波,
陈龙鑫,
刘斌琨,
朱慧娟,
许镇义,
and 柏鹏
[Abs]
本申请公开了一种故障诊断模型的训练方 法、装置、电子设备及存储介质,属于计算机技术 领域。所述方法包括:获取多个工况中各工况集 下的故障数据,分别作为源域数据,并获取目标 工况下的故障数据,作为目标域数据,所述工况 集包括所述多个工况中的至少一个工况,所述工 况集下的故障数据为已标记故障类别的数据,所 述目标工况下的故障数据为未标记故障类别的 数据;确定所述目标域数据与各所述源域数据之 间的目标分布差异;根据所述目标分布差异选取 源域数据作为训练数据;根据所述训练数据,对 所述目标工况的故障诊断模型进行训练。本申请 能够提高模型训练效果,进而提高对目标工况进 行故障诊断的准确率。
-
产线设备故障预测方法、装置、电子设备及存储介质
赵云波,
董少杰,
刘斌琨,
朱慧娟,
许镇义,
and 柏鹏
[Abs]
本申请公开了一种产线设备故障预测方法、 装置、电子设备及存储介质,属于计算机技术领 域。所述方法包括:获取测试结果序列,测试结果 序列包括多个电路板的测试结果信息,测试结果 信息包括电路板中各功能模块的测试结果;根据 测试结果序列,获取各功能模块的良率序列;根 据各功能模块的良率序列,获取多个各功能模块 中的目标功能模块与其他功能模块的相关性序 列;根据各功能模块的良率序列和相关性序列, 获取目标功能模块的良率预测信息;根据相关性 序列和良率预测信息,预测目标功能模块对应的 产线设备是否存在故障。本申请能够准确预测产 线设备是否出现故障,以及时发现故障设备,避 免生产事故的发生。
Journal Articles
-
Cross-Sensor Generative Self-Supervised Learning Network for Fault Detection Under Few Sample
Huijuan Zhu,
Yun-Bo Zhao ,
Xiaohui Yan,
Yu Kang,
and Binkun Liu
Journal of Systems Science & Complexity
2025
[Abs]
[pdf]
In this paper, a cross-sensor generative self-supervised learning network is proposed for fault detection of multi-sensor. By modeling the sensor signals in multiple dimensions to achieve correlation information mining between channels to deal with the pretext task, the shared features between multisensor data can be captured, and the gap between channel data features will be reduced. Meanwhile, in order to model fault features in the downstream task, the salience module is developed to optimize cross-sensor data features based on a small amount of labeled data to make warning feature information prominent for improving the separator accuracy. Finally, experimental results on the public datasets FEMTO-ST dataset and the private datasets SMT shock absorber dataset(SMT-SA dataset) show that the proposed method performs favorably against other STATE-of-the-art methods.
Conference Articles
-
A Reliable Ensemble Model Based on Hierarchical Component Features for Repair Label Prediction of Soldering Defects
Longxin Chen,
Yunbo Zhao,
Binkun Liu,
Shaojie Dong,
Huijuan Zhu,
and Peng Bai
In 2024 14th Asian Control Conference (ASCC)
2024
[Abs]
[pdf]
Using solder paste inspection (SPI) and automated optical inspection (AOI) data, accurate prediction for repair labels of soldering defective printed circuit board (PCB) components can help reduce labor costs. Existing research tries to pick out both the false defect components (actually good) and impossible-to-repair components among defective PCB components, using SPI and AOI data. However, it is inappropriate to pick out the false defect components from screened components using defective information in AOI data. Therefore, the problem setting of existing research is inappropriate, resulting in the algorithm’s performance not meeting actual requirements. To address this problem, we only care about the reliable prediction of impossible-to-repair components. We propose a hierarchical component feature extraction method that can comprehensively characterize the degree of component defects from multiple levels, including pin level and component level. Then we apply the ensemble model based on XGBoost and TabNet and adjust the probability threshold of components judged as impossible-to-repair category, achieving the reliable prediction of impossible-to-repair components. Finally, we validated our method on real datasets and achieved better experimental results compared to baseline methods, which can meet actual requirements,
-
Anomaly Detection for Surface of Laptop Computer Based on Patchcore Gan Algorithm
Huijuan Zhu,
Yu Kang,
Yun-Bo Zhao ,
Xiaohui Yan,
and Junqiang Zhang
In 2022 41st Chinese Control Conference (CCC)
2022
[Abs]
[doi]
[pdf]
Timely detection of notebook appearance defects is an important means to prevent products from being delivered to customers before leaving the factory.In industrial production, more emphasis is placed on fast and accurate detection methods, but the existing difficulties: 1. Defect samples are rare and difficult to obtain; 2. In high-resolution images, there are slight differences between abnormal samples and normal samples; 3. Slowly detection and insufficient accuracy.The existing methods mainly use a large amount of abnormal samples, so it is difficult to extend to the field of notebook appearance anomaly detection.To solve this problem, we designed a method that firstly uses unsupervised PatchCore which the algorithm was trained on normal samples and Defect GAN is used in test phase. To create a large number of verisimilitude abnormal samples and test these samples with PatchCore. On TKP-Surface datasets, the AUROC score of image-level anomaly detection achieves 96.1%, which meets the requirements of industrial applications.
学位论文
Theses
-
减震器的数据扩充和跨传感器故障检测方法研究
朱慧娟
安徽大学, 合肥
2024
[Abs]
[pdf]
减震器在表面贴装技术中扮演着至关重要的角色。它通过减少锡膏印刷机的震动 来确保锡膏印刷质量,从而间接影响了后续整体流程的顺利进行。虽然在生产线上减震 器的故障较少发生,但一旦发生故障,往往很难及时检测和排查出来,将导致整个工厂 停工数小时进行检修,给生产带来严重的影响和经济损失。在这个背景下,对减震器进 行故障检测成为确保设备在故障发生时能够及时预警的关键步骤。故障检测的重要性 体现在以下几个方面:及时识别设备异常运行,使得问题可以尽早得到解决;加速维护 和降低停机时间,以最小化生产中断的影响;同时,故障检测也有助于确保生产的安全 性。因此,对减震器进行故障检测,可以提高生产线的稳定性和效率,减少停机时间和 经济损失,从而对整体生产过程产生重要的影响。 目前,对减震器的故障检测研究存在一些局限性,这些局限性主要包括以下方面: 首先,减震器数据的稀缺和不平衡导致故障检测模型的准确率不尽如人意,进而影响了 模型在实际应用中的效果。其次,现有的模型未能充分考虑多传感器数据之间的显著差 异特点,这些数据通常以堆叠的方式输入网络进行模型训练,这种方法不利于模型捕捉 多传感器变量之间的共享特征,也难以减小变量数据特征之间的差距,因此对于提升模 型性能存在一定的制约。 为了克服上述问题,本文着重研究了减震器故障检测问题,并在以下几个方面做出 了主要贡献: 1、针对减震器数据集稀少和类别不平衡的问题,提出了注意力生成对抗网络,生 成了高仿真的减震器数数据集。该方法主要通过多生成器结构来生成数据,保证了跨传 感器各数据特征组内的相关性,并利用注意力机制捕获数据的时序性并修正各特征间 的相关性。该方法提出了分组对抗生成的设计,从而生成高度贴合真实样本的生成数 据。针对工业场景下有效标注稀缺的问题,该方法可以有效建模数据分布,通过拓充生 成大量真实的减震器故障数据,使减震器故障检测模型更好的学习故障数据的特征,从 而提高模型的检测性能。 2、针对减震器多传感器的特点,提出了一种跨传感器多层级的生成式自监督学习 网络用于故障检测。针对具有大量无标注数据的场景,我们设计了多层级自编码器结 构来进行表征学习,通过在多个维度中建模传感器信号,以实现特征之间的相关信息挖掘,从而有效提升特征的表达能力。同时,我们在下游缺陷预测任务中微调任务重引入 了显著性模块,使警告特征信息突出,从而提高分类器的准确性。在减震器数据集上进 行的实验结果表明,所提出的方法在性能方面显著优于其他先进方法。 本文重点关注了减震器数据集的数据不平衡特性以及多传感器数据的特性,分别提 出了注意力生成式对抗网络和跨传感器多层级的自监督学习网络,为以上问题提供了 有效的解决方案,提高了故障检测性能,对智能制造的故障检测推进做出了一定贡献。
毕业去向
新大陆数字技术股份有限公司, 算法工程师
外部链接
个人博客