个人信息
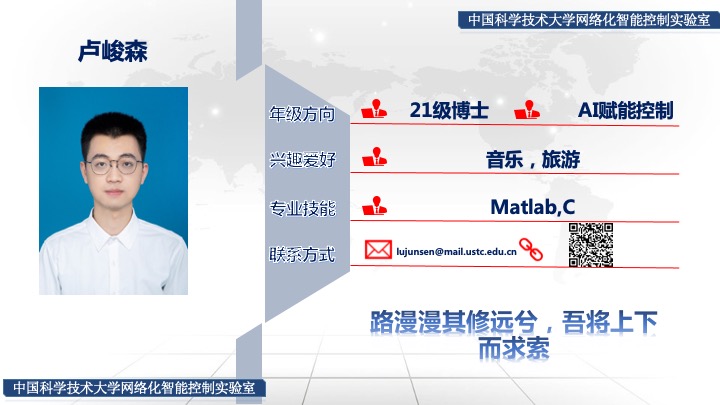
参与实验室科研项目
人机混合智能系统双层智能测试评估技术研究
复杂环境下非完全信息博弈决策的智能基础模型研究
机载座舱智能系统人机信任动态演化建模方法研究
学术成果
共撰写/参与撰写专利 0 项,录用/发表论文 4 篇,投出待录用论文1篇。
Book Chapters
-
Trust-Modulated Authority Allocation in Human-Guided Goal Recognition Tasks
Ruiyu Xia,
Yunbo Zhao,
Junsen Lu,
Yang Wang,
Pengfei Li,
and Yu Kang
In Frontiers in Artificial Intelligence and Applications
2025
[Abs]
[doi]
[pdf]
In shared control teleoperation, the machine infers the humans’ goal to provide effective assistance, which we call human-guided goal recognition. However, current methods mainly use algorithm confidence to assign control authority during the process, which makes it difficult to correct machine inference errors under high confidence. To address this problem, we propose a trust model that considers machine capability fluctuations and human-machine interaction experience. We also add trust as a dynamic assessment of machine capabilities to authority allocation to improve the success rate of the tasks. Finally, we verify the effectiveness of the proposed method through experiments.
Conference Articles
-
A Human-Machine Trust Model and Trust Calibration Method for Decision-aid Systems
Xiaojun Zhu,
Lunfei Liang,
Junsen Lu,
Yun-Bo Zhao ,
and Yu Kang
In The 44th Chinese Control Conference (CCC 2025)
2025
[Abs]
[pdf]
With the development of AI technology, decision-aid systems are widely applied. The trust level between human and decision aids has a great impact on the overall performance of the system. Current research on human-machine trust has deficiencies in trust modeling and trust calibration, especially lacking quantitative characterization of human trust level. In response to this, this paper proposes a human-machine trust evaluation model and a transparency-based trust calibration method for decision aid systems. Firstly, a dynamic evolution model of human-machine trust is constructed, with machine performance and past trust as influencing factors. Secondly, the meaning of trust calibration is elaborated, the impact of transparency on the trust model is analyzed, and a transparency-based trust calibration method is proposed. Through the simulation experiment of a tumor prediction medical assistance system, the rationality of the proposed trust model and the effectiveness of the calibration method are verified.
-
A Human-Machine Trust Model Integrating Machine Estimated Performance
Shaojun Chen,
Yun-Bo Zhao ,
Yang Wang,
and Junsen Lu
In 2023 6th International Symposium on Autonomous Systems (ISAS)
2023
[Abs]
[doi]
[pdf]
The prediction of human trust in machines within decision-aid systems is crucial for improving system performance. However, previous studies have only measured machine performance based on its decision history, failing to account for the machine’s current decision state. This delay in evaluating machine performance can result in biased trust predictions, making it challenging to enhance the overall performance of the human-machine system. To address this issue, this paper proposes incorporating machine estimated performance scores into a human-machine trust prediction model to improve trust prediction accuracy and system performance. We also provide an explanation for how this model can enhance system performance. To estimate the accuracy of the machine’s current decision, we employ the KNN method and obtain a corresponding performance score. Next, we report the estimated score to humans through the human-machine interaction interface and obtain human trust via trust self-reporting. Finally, we fit the trust prediction model parameters using data and evaluate the model’s efficacy through simulation on a public dataset. Our ablation experiments show that the model reduces trust prediction bias by 3.6% and significantly enhances the overall accuracy of humanmachine decision-making.
-
Strategy Generation Based on DDPG with Prioritized Experience Replay for UCAV
Junsen Lu,
Yun-Bo Zhao ,
Yu Kang,
Yuhui Wang,
and Yimin Deng
In 2022 International Conference on Advanced Robotics and Mechatronics (ICARM)
2022
[Abs]
[doi]
[pdf]
Unmanned combat aerial vehicles are becoming essential participants in future air-combat scenarios, while the optimal control strategy remains a great challenge due to the high dynamics of the aerial vehicles themselves as well as the environmental uncertainties in air-combat. Based on a deep deterministic policy gradient algorithm framework, an air combat decision-making strategy is designed and implemented, and further a prioritized experience replay method is proposed for the proposed algorithm to further improve the efficiency in the training process. Simulation experiments show that, at much reduced training cost, the proposed approach achieves superior air combat performance with fast convergence.