个人信息
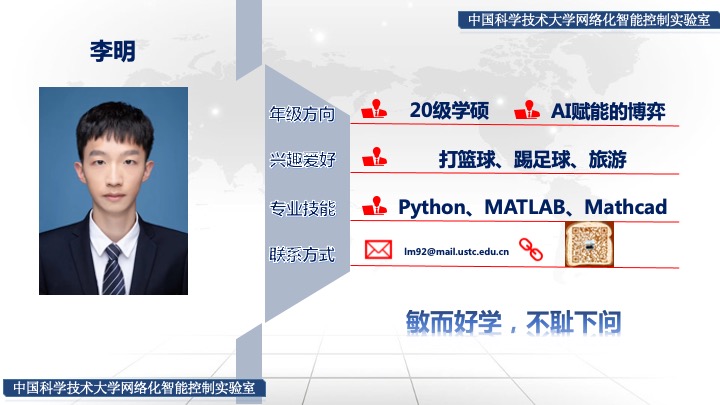
参与实验室科研项目
复杂环境下非完全信息博弈决策的智能基础模型研究
研究课题
针对不确定复杂环境下多群体博弈决策中的瓶颈问题,围绕其非完全信息、高智能、强动态的特点,从智能模型构建、多群体博弈决策理论形成以及人机对抗性能验证与评估等层面开展研究。
学术成果
共撰写/参与撰写专利 0 项,录用/发表论文 2 篇,投出待录用论文0篇。 联培学生可能有其他不在此展示的论文/专利。
Journal Articles
-
Autonomous Multi-Drone Racing Method Based on Deep Reinforcement Learning
Yu Kang,
Ming Li,
and Yun-Bo Zhao
Sci. CHINA Inf. Sci.
2024
[Abs]
[pdf]
Racing drones have attracted increasing attention due to their remarkable high speed and excellent maneuverability. However, Autonomous multi-drone racing is quite difficult since it calls for quick and dexterous drone flight in intricate surroundings and rich drone interaction. To address these issues, we propose a novel autonomous multi-drone racing method based on deep reinforcement learning. A new set of reward functions is proposed to make racing drones learn the racing skills of human experts. Unlike previous methods that require global information about track and track boundary constraints, the proposed method requires only limited localized track information within the range of its own on-board sensors. Further, the dynamic response characteristics of racing drones are incorporated into the training environment, so that the proposed method is more in line with the requirements of real drone racing scenarios. In addition, our method has low computational cost and can meet the requirements of real-time racing. Finally, the effectiveness and superiority of the proposed method are verified by extensive comparison with the state-of-the-art methods in simulation and in the physical world.
Conference Articles
-
Shared Autonomy Based on Human-in-the-loop Reinforcement Learning with Policy Constraints
Ming Li,
Yu Kang,
Yun-Bo Zhao ,
Jin Zhu,
and Shiyi You
In 2022 41st Chin. Control Conf. CCC
2022
[Abs]
[doi]
[pdf]
In shared autonomous systems, humans and agents cooperate to complete tasks. Since reinforcement learning enables agents to train good policies through trial and error without knowing the dynamic model of the environment, it has been well applied in shared autonomous systems. After inferring the target from human inputs, agents trained by RL can accurately act accordingly. However, existing methods of this kind have big problems: the training of reinforcement learning algorithms require lots of exploration, which is time-consuming, lack of security guarantee and likely to cause great losses in the training process. Moreover, most of shared control methods are human-oriented, and do not consider the situation that humans may make wrong actions. In view of the above problems, this paper proposes human-in-the-loop reinforcement learning with policy constraints. In the training process, human prior knowledge is used to constrain the exploration of agents to achieve fast and efficient learning. In the process of testing we incorporate policy constraints in the arbitration to avoid serious consequences caused by human mistakes.
学位论文
Theses
-
基于训练和执行双阶段联合设计的人机智能决策方法研究
李明
中国科学技术大学, 合肥
2023
[Abs]
[pdf]
在人机混合智能系统中,人工智能赋能的机器智能和人类智能相互融合,在 特定场景下可以超越单独人类或者机器的决策性能,成为当前的研究热点。但 是,与传统的人机系统和人工智能算法不同,人机混合智能系统的决策效果不仅 受到训练阶段人工智能算法性能的影响,比如算法的泛化性和鲁棒性,而且也会 受到执行阶段人类和机器决策混合方法的影响,比如人类和机器控制权的分配。 如何从整体上优化人机混合智能系统的决策性能,是当下重要的研究课题。 本文面向深度强化学习算法驱动的人机混合智能决策系统的序贯决策问题, 同时从算法的训练端和执行端出发,通过引入人类智能的方式提高系统决策的 鲁棒性和安全性,最终提高人机混合智能系统的决策性能。本文工作主要包含以 下三个方面: (1) 针对强化学习算法驱动的人机共享控制系统的序贯决策问题,在训练阶 段提出了基于人类策略限制下人在环上强化学习算法,避免机器做出危险的行 为,同时提高了算法的采样效率;在执行阶段提出了包含人类决策评估的仲裁机 制,舍弃了人类错误的决策,提高了系统的整体性能。实验结果表明,此方法成 功提高了算法训练的采样效率和系统执行任务的成功率。 (2) 针对多机竞速场景下强化学习算法驱动的人机介入控制系统的序贯决策 问题,在训练阶段引入了包含人类反馈奖励的奖励函数组,以引导机器理解竞速 规则,减少了执行阶段人类的介入次数;在执行阶段引入了人类的两级介入机 制,避免违背规则或者容易造成事故的行为出现,同时降低了人类介入时的操作 负担。实验结果表明,此方法缩短了无人机的单圈耗时,提高了系统决策的安全 裕度,并且减轻了人类的介入负担。 (3) 针对上述人机混合序贯决策方法,本文以旋翼无人机为背景,搭建了从 仿真到现实的人机实验平台,提出了算法部署到真实物理场景的整体流程和框 架,并针对提出的多机竞速场景下强化学习算法驱动的人机介入控制方法,进行 了现实场景下的算法验证。
毕业去向
杭州同花顺智能科技有限公司, 量化研究员