个人信息
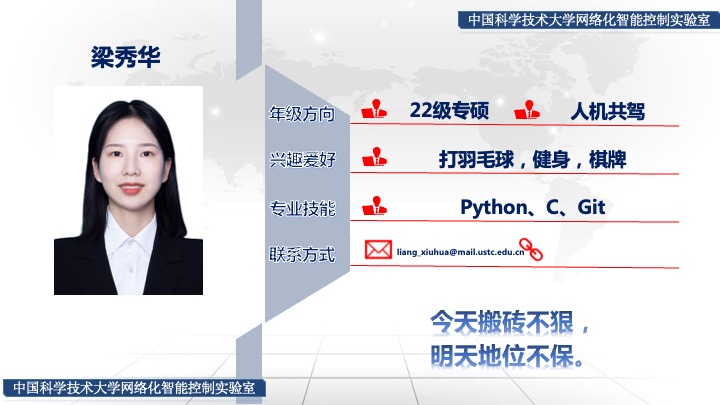
参与实验室科研项目
人机智能协同关键技术及其在智能制造中的应用
基于数据驱动和联合设计的无线网络化控制系统的使能建模和设计
研究课题
基于长时域模型的人机共驾共享控制策略研究
学术成果
共撰写/参与撰写专利 0 项,录用/发表论文 2 篇,投出待录用论文0篇。 联培学生可能有其他不在此展示的论文/专利。
Conference Articles
-
An Authority Allocation Strategy for Shared Control in Human-Machine Cooperative Driving via Lane-Based Probabilistic Collision Risk Assessment
Xiuhua Liang,
Yunbo Zhao,
Yu Kang,
and Chang Xu
In 2024 14th Asian Control Conference (ASCC)
2024
[Abs]
[pdf]
An effective shared control strategy plays a crucial role in assisting drivers during hazardous situations in human-machine cooperative driving. This study introduces an author-ity allocation strategy for shared control based on collision risk assessment in a long-term maneuver. Initially, maneuvers are categorized by lanes, which can properly represent lane-based driving characteristics in real-world driving conditions. Subsequently, multiple lane models are built to combine with interactive multiple models to compute target lane probabilities. The target lane probabilities indicate the likelihood of a vehi-cle moving toward or remaining in each lane, determined by its lateral position in curvilinear coordinates. Finally, collision risk is evaluated through the integration of model probability distribution of lanes and artificial potential field value between a pair of predicted trajectories. Intuitively, the driver’s authority is directly correlated with the collision risk, so authorities between the driver and the machine are determined by the collision risk through a piecewise proportional function. The effectiveness of the proposed algorithm is verified using the Carla simulator in a cut-in scenario.
-
A Novel Haptic Takeover Method Based on Human-Machine Collaboration States
Yunbo Zhao,
Zuhao Xie,
Chang Xu,
Xiuhua Liang,
Ruiyu Xia,
and Jiayu Li
In 2024 14th Asian Control Conference (ASCC)
2024
[Abs]
[pdf]
The current stage of autonomous driving calls for drivers to remain actively engaged within the control loop in anticipation of the need for takeover operations. However, regaining control of the vehicle from a state of low situation awareness (SA) poses a challenge. To address this issue, this research introduces a novel takeover method based on haptic shared control, ensuring a smooth and safe takeover process. A symmetric softmax function is formulated to evaluate muscle state, taking into account the varying torque thresholds associated with different vehicle speeds, as well as utilizing the driver’s cognitive state to characterize their SA. Within the takeover process, a coordinator leverages the driver’s SA and human-machine intention similarity to determine the current state of human-machine collaboration. Subsequently, different control allocation strategies are then adopted for different states, and the driver is guided through force feedback. Experiment results demonstrate the effectiveness of the proposed method, showcasing its ability to facilitate a smooth and safe transfer of control, regardless of the presence of conflicts or the harmonious state existing between the human driver and the automated system.
博客文章