个人信息
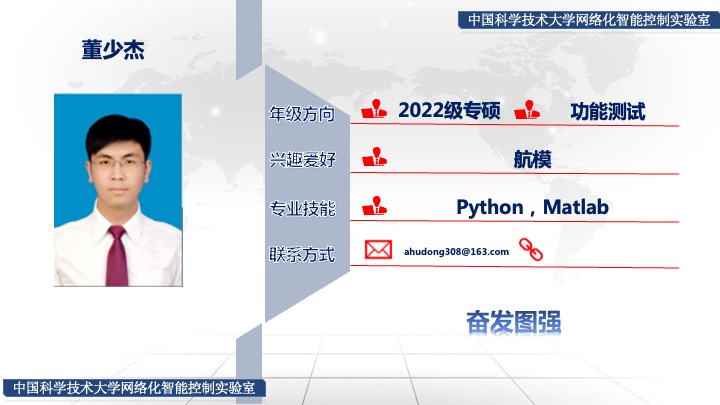
参与实验室科研项目
人机智能协同关键技术及其在智能制造中的应用
非可信智能驱动的可靠智造
研究课题
基于时序预测的电路板功能测试优化策略及其验证
学术成果
共撰写/参与撰写专利 1 项,录用/发表论文 3 篇,投出待录用论文1篇。
patent
-
产线设备故障预测方法、装置、电子设备及存储介质
赵云波,
董少杰,
刘斌琨,
朱慧娟,
许镇义,
and 柏鹏
[Abs]
本申请公开了一种产线设备故障预测方法、 装置、电子设备及存储介质,属于计算机技术领 域。所述方法包括:获取测试结果序列,测试结果 序列包括多个电路板的测试结果信息,测试结果 信息包括电路板中各功能模块的测试结果;根据 测试结果序列,获取各功能模块的良率序列;根 据各功能模块的良率序列,获取多个各功能模块 中的目标功能模块与其他功能模块的相关性序 列;根据各功能模块的良率序列和相关性序列, 获取目标功能模块的良率预测信息;根据相关性 序列和良率预测信息,预测目标功能模块对应的 产线设备是否存在故障。本申请能够准确预测产 线设备是否出现故障,以及时发现故障设备,避 免生产事故的发生。
Journal Articles
-
Modelling and Optimizing Motherboard Functional Testing in Laptop Manufacturing
Peng Bai,
Yu Kang,
Kangcheng Wang,
Yunbo Zhao,
and Shaojie Dong
J Syst Sci Complex
2024
[doi]
[pdf]
Conference Articles
-
A Reliable Ensemble Model Based on Hierarchical Component Features for Repair Label Prediction of Soldering Defects
Longxin Chen,
Yunbo Zhao,
Binkun Liu,
Shaojie Dong,
Huijuan Zhu,
and Peng Bai
In 2024 14th Asian Control Conf. ASCC
2024
[Abs]
[pdf]
Using solder paste inspection (SPI) and automated optical inspection (AOI) data, accurate prediction for repair labels of soldering defective printed circuit board (PCB) components can help reduce labor costs. Existing research tries to pick out both the false defect components (actually good) and impossible-to-repair components among defective PCB components, using SPI and AOI data. However, it is inappropriate to pick out the false defect components from screened components using defective information in AOI data. Therefore, the problem setting of existing research is inappropriate, resulting in the algorithm’s performance not meeting actual requirements. To address this problem, we only care about the reliable prediction of impossible-to-repair components. We propose a hierarchical component feature extraction method that can comprehensively characterize the degree of component defects from multiple levels, including pin level and component level. Then we apply the ensemble model based on XGBoost and TabNet and adjust the probability threshold of components judged as impossible-to-repair category, achieving the reliable prediction of impossible-to-repair components. Finally, we validated our method on real datasets and achieved better experimental results compared to baseline methods, which can meet actual requirements,
-
Prediction of Yield in Functional Testing of Motherboards in Laptop Manufacturing
Yunbo Zhao,
Shaojie Dong,
Yu Kang,
Kangcheng Wang,
Longxin Chen,
and Peng Bai
In 2024 14th Asian Control Conf. ASCC
2024
[Abs]
[pdf]
Functional testing stands as a pivotal quality control step in the production process of laptop motherboards, aiming to validate the proper functioning of various components. However, due to the multitude of functional modules involved on the motherboard, testing all of them requires a significant amount of time and resources. As a result, production line engineers often rely on empirical selection of modules with low yield rates for testing. However, such empirical yield estimation is often inaccurate. To address this challenge, this study proposes a hybrid model based on XGBoost and Long Short-Term Memory (LSTM) networks to predict the yield of each functional module. By harnessing the feature learning capability of XGBoost and the sequential modeling power of LSTM, this model efficiently explores the intricate correlations among motherboard functional modules, thereby accurately forecasting their yields. We extensively train and validate the model using historical production data and successfully deploy it on real laptop motherboard production lines. Experimental results demonstrate that our hybrid model accurately predicts the yield of each functional module, providing crucial guidance for the functional testing process. Through in-depth analysis of the predicted yield results, engineers can systematically choose testing projects to save time and resources. This research offers a novel approach and pathway for enhancing motherboard production efficiency and quality.
博客文章